Process of Mobility: Scenario

The Process of Mobility consists of the six steps performed by a vehicle traversing through space: Scenario, Sense, Perceive, Plan, Control, and Response. The continuous and cyclic manner of the steps creates the Process of Mobility. Historically, these 6 steps were conducted by humans, but automated driving systems (ADS) allow these steps to be supplemented (and increasingly supplanted).
The scenario step is the start of the entire process, like the first domino in the chain reaction. Errors in this step can lead to the remaining steps being inaccurate. Scenario is a combination of the operational driving domain (ODD) and the surrounding actors (e.g., other vehicles, pedestrians), both dynamic and static. For many years, companies optimized vehicle ODDs based on regulatory requirements and evaluation methods, as well as some public evaluations enabling easier engineering practices. As vehicles become more automated, the ODD has expanded well beyond these typical scenarios.
Regulatory requirements are intended to enhance the safety and reliability of vehicles. These requirements are developed from examining crashes and reported issues with vehicles from the public. Using this data, specific use cases are identified, testing methods are developed, and then regulations are implemented. Use cases change as the vehicle capabilities change. For example, early vehicles had limited power and speed, and had to navigate through conditions that primarily included horses, pedestrians, and bicycles. These conditions did not require side impact crash testing or high-speed stability consideration because vehicles never encountered those situations. Combined with few vehicles on the road, crash worthiness was not high on priority. But as vehicles speeds increased and encountered more vehicles on the road, the ODD changed. Crashworthiness became increasingly important, and the first crash test was conducted by General Motors in 1934, and other safety technologies were implemented.
Another scenario example is the double lane change from the Federal Motor Vehicle Safety Standard (FMVSS) 126. This is a dynamic test to check the chassis control functionality. The Chassis Control feature attempts to keep the vehicle from rotating too much or rolling over. The FMVSS 126 test was developed around general test parameters based on the vehicle, without consideration of surrounding actors. Multiple original equipment manufacturers came together to develop the methodology for the variety of vehicles on the road and then worked with the National Highway Traffic Safety Administration (NHTSA) to define the regulations. This can be tested in a couple days for each configuration, controls updated if needed, and then tested again.
The great thing about the regulatory tests is they can be easily replicated in fairly well controlled environments for physical testing, increasing the repeatability of the tests. Engineers love repeatability for a performance targets. But as the model variations expand, physically testing all the variations is impossible. This is when simulation becomes critically important. The practice of simulation has been conducted for many years to assess vehicle variations in specific scenarios. The examples discussed above are now mainly conducted using simulations to reduce cost and time. The use of simulations is enabled because the inputs are known, and the response can be easily quantified i.e., lateral displacement during FMVSS 126.
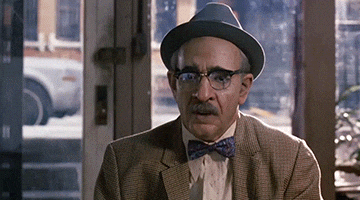
In the case of ADS vehicles, the ODD is expanded to enormous levels of potential scenarios. Many argue that physically evaluating an ADS for all the scenarios is unrealistic. This is primarily due to the vast range of scenarios that people encounter every day on the road that the ADS is now expected to navigate. With the expansion of the ODD, simulation is now used for modeling the scenarios themselves along with the vehicle technology. This is really a necessity since ADS vehicles deal with every scenario (known and unknown) not just specific use cases defined by regulations. But what scenarios should be prioritized? Should the scenarios come from an industry standard set, the public, or an engineer’s imagination? Establishing scenarios based on real-world conditions is the best basis for simulations. How to obtain real-world scenarios is the next hurdle.
The Virginia Tech Transportation Institute (VTTI) has 70 million Miles of Naturalistic Driving Data (NDD), which is data collected using video and sensors to capture this real-world data without interfering with the driver. Did you just have an Aha moment? Seventy Million miles is a huge pool of data, and researchers at VTTI have been studying human behaviors in all kinds of scenarios. They can tell you how often and how long people turn their heads to look at an accident. They can tell you how often people cut off tractor trailers. We can quantify how many times a driver encounters a deer crossing the road on I-81. With seventy million miles (which keeps growing every day), they can provide the most relevant set of scenarios per ODD, the frequency of those scenarios, and what the humans did in those scenarios (more on this in our future Response blog, stay tuned).

This is where GCAPS’ expertise on physical to virtual transformation and simulation come in. Utilizing VTTI’s 70 million miles of NDD, GCAPS produces simulation ready data sets that represent the range of scenarios an ADS may encounter- normal driving, near crash, and crash scenarios. The replication of the scenarios includes all dynamic actors, static actors, and terrain. These data sets can be applied to many of the simulation software tools being used in industry, as GCAPS utilizes a tool agnostic approach. With these data sets, one can virtually test the ADS to compare it to the human response. Pairing that with the statistical prowess of the VTTI researchers, one can properly focus on which events happen more often than others. This allows researchers to test events based on real-life frequency.
While thousands of scenarios have been captured using NDD, every possible scenario has not been recorded. To aid in this gap, scenario variations are created. Creating variations are done in a methodical way, not simply simulating a vehicle going 100 mph on a city block. Simulation Ready Data sets are produced in an OPENSCENARIO format, one of the most common formats used for scenario description. Remember, engineers like repeatable processes for development, and everyone using the same terms and conditions for scenarios is vitally important. When Simulation Ready Data sets are in the OPENSCENARIO format, variations can be quickly created. The variations and scenarios are selected by GCAPS using intelligent sampling and variation. Scenarios are mapped into a parameter space, which consists of the parameters influencing the scenario. The influencing parameters are ones that have a noticeable effect on the scenario, which can be identified based on a knowledge-based process and/or a statistical approach. Moreover, the distribution of NDD scenarios over the parameter space is associated with a certain probability of occurrence, which needs to be considered during test case selection process. It is important to keep in mind an ADS vehicle can behave differently from human driving patterns. Researchers use a knowledge-based process to adjust the scenarios with ADS driving behavior. For instance, the value range of some parameters could be modified to address a mismatch between human and ADS driving pattern.

With many thousands of base scenarios and variations, do we need to run them all? GCAPS uses a mixture of sampling techniques to maximize the test coverage and keep the number suitable for a practical testing procedure. Random, systematic probability, or purposive non-probability sampling methods can be used to focus on representative and safety critical events. To perform the purposive sampling, selected events should meet certain criteria. For example, event duration, time to collision or gap of a selected event need to be greater or smaller than a certain value. This sampling and variation techniques allows researchers to pay attention to safety critical scenarios during the testing procedures, which is an important step in the ADS verification process. Utilizing the Intelligent Sampling and Variation approach, GCAPS can turn one scenario into many others which are plausible and down selected to create an efficient test plan.
Since Scenario is the first of a number of steps in the Process of Mobility, defining the ODD, the relevant actors, and proper replication are critically important. If the scenario step is misidentified, then all the remaining dominos fall the wrong way for getting the proper response from the system, as they are reliant upon the scenario step. The work we do at GCAPS ensures the scenarios are robust, and we provide a strong foundation upon which the other steps in the Process of Mobility occur. Stay tuned for more information about the Sense step.